Introduction to Artificial Intelligence
Defining Artificial Intelligence
Common Misconceptions About AI
Brief History of Artificial Intelligence
Early Beginnings and Theoretical Foundations
The concept of machines exhibiting intelligence dates back to ancient times, but the formal foundation of AI was laid in the 20th century. The term “Artificial Intelligence” was first coined in 1956 at the Dartmouth Conference by John McCarthy, who is considered one of the founding fathers of AI. Early AI research focused on problem-solving and symbolic methods, laying the groundwork for more sophisticated technologies.
The AI Winters and Resurgence
AI has experienced periods of intense progress, followed by “AI winters”—times when interest and funding waned due to unmet expectations. The 1970s and 1980s saw such winters, but the resurgence began in the 1990s with advancements in machine learning, data processing power, and the internet. The current era of AI is marked by rapid developments and significant breakthroughs, particularly in machine learning and deep learning.
Modern AI: Breakthroughs and Application
Today’s AI is far more advanced, driven by big data, powerful computing capabilities, and sophisticated algorithms. Breakthroughs in AI include natural language processing, computer vision, and reinforcement learning. These advancements have led to practical applications in numerous fields, from healthcare and finance to entertainment and customer service.
How Artificial Intelligence Works
Core Components of AI
Machine Learning (ML)
Machine learning is a subset of AI that focuses on developing algorithms that allow computers to learn from and make predictions or decisions based on data. Unlike traditional programming, where specific rules and instructions are coded, machine learning enables systems to improve their performance over time by identifying patterns in data.
Supervised Learning
Supervised learning is the most common type of machine learning. It involves training an algorithm on a labeled dataset, where the correct output is provided for each input. The algorithm learns by comparing its predictions to the actual outcomes, adjusting until it can make accurate predictions on new, unseen data. This method is widely used in applications like spam detection, image recognition, and medical diagnostics.
Unsupervised Learning
In unsupervised learning, the algorithm is given data without explicit instructions on what to do with it. The goal is for the system to identify hidden patterns or groupings within the data. This type of learning is often used in clustering tasks, such as market segmentation, where companies want to identify distinct groups of customers based on purchasing behavior.
Reinforcement Learning
Reinforcement learning involves training an AI model to make decisions by rewarding it for correct actions and penalizing it for incorrect ones. Over time, the model learns to maximize its rewards, improving its decision-making process. This approach is commonly used in robotics, gaming, and autonomous vehicles, where the AI must navigate complex environments.
Neural Networks and Deep Learning
What Are Neural Networks?
Introduction to Deep Learning
Deep learning takes neural networks to the next level by increasing the number of layers, which allows the network to learn and model complex patterns in large datasets. Deep learning is responsible for many recent AI advancements, including image and speech recognition, language translation, and even creative applications like generating art and music.
Natural Language Processing (NLP)
How NLP Understands Human Language
Common Applications of NLP
Computer Vision
Basics of Computer Vision
Computer vision is another crucial component of AI, enabling machines to interpret and make decisions based on visual data. This technology involves teaching computers to “see” and process images or videos similarly to how humans do. It combines machine learning with image processing to identify objects, recognize patterns, and even predict actions.
Applications in Real World
Computer vision is used in a wide range of applications. In healthcare, it helps in diagnosing diseases by analyzing medical images like X-rays or MRIs. In security, it’s used for facial recognition and surveillance systems. In everyday life, computer vision powers features like facial recognition in smartphones and automated tagging in social media photos.
AI in Everyday Life
AI in Smartphones
AI in Healthcare
AI in Transportation
AI in Customer Service
Ethical Considerations in AI
The Debate on AI Bias
AI and Privacy Concerns
AI in Decision Making: Risks and Benefits
The Future of Artificial Intelligence
Predictions for AI Development
Potential Impact on Various Industries
The Role of AI in Solving Global Challenges
FAQs about Artificial Intelligence
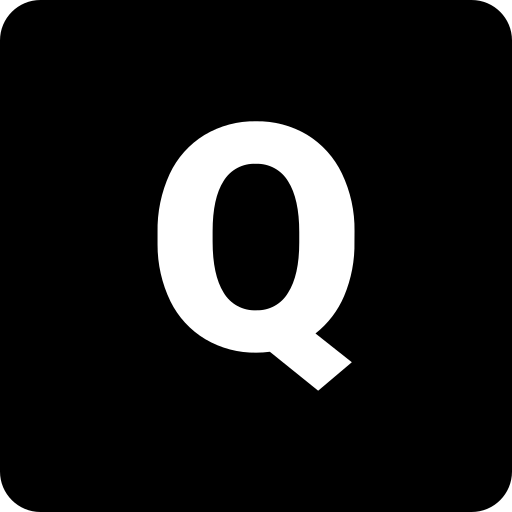
What is the difference between AI and Machine Learning?
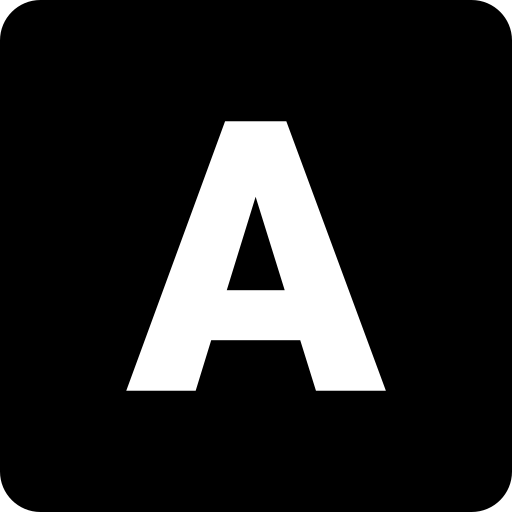
AI is the broader concept of machines being able to carry out tasks in a way that we would consider "smart." Machine learning is a subset of AI that focuses specifically on the ability of machines to learn from data and improve over time.
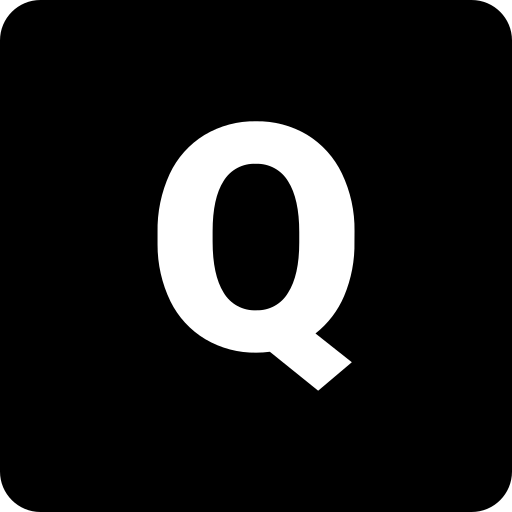
Can AI completely replace human jobs?
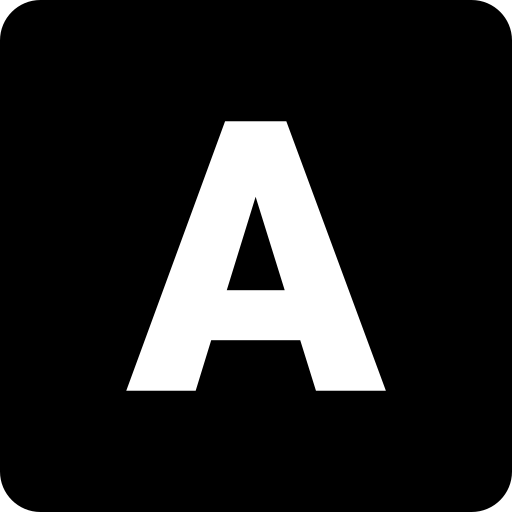
While AI is likely to automate certain tasks and jobs, it is also expected to create new opportunities. The key will be in reskilling the workforce and preparing for changes in the job market. Some roles may disappear, but others will emerge that require human creativity, empathy, and problem-solving skills.
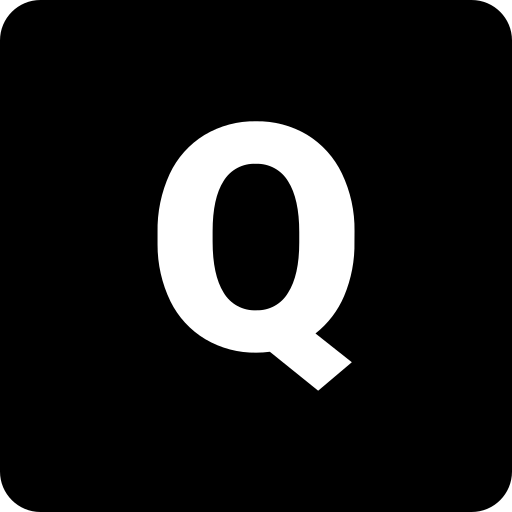
How safe is AI in today's world
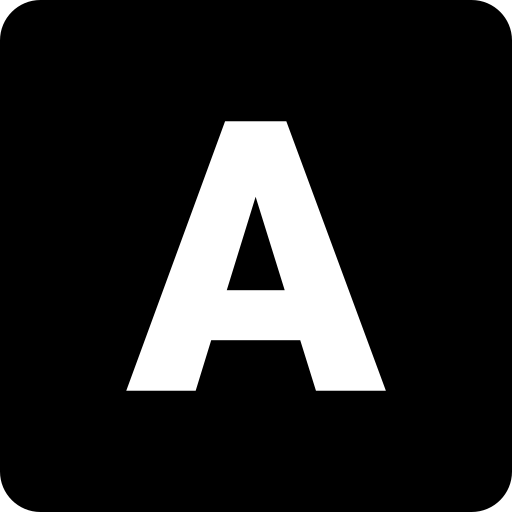
AI safety is an ongoing concern, particularly in areas like autonomous vehicles and security. While AI systems are designed with safety in mind, unexpected outcomes or malicious uses can pose risks. Ongoing research and regulation are necessary to ensure AI systems are safe and beneficial.
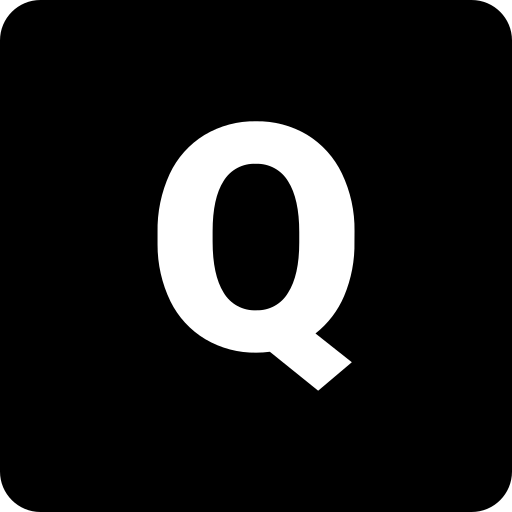
Is AI only for big tech companies?
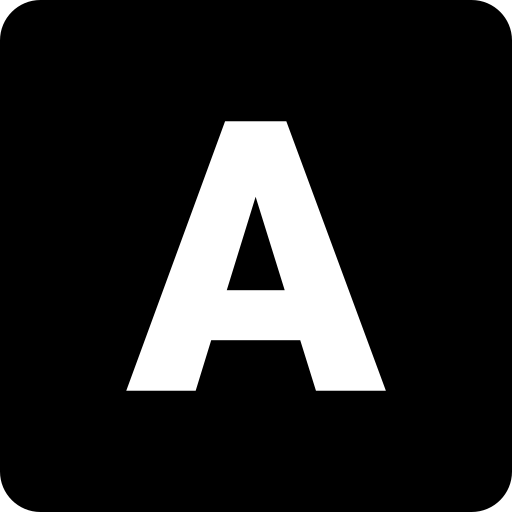
No, AI is increasingly accessible to businesses of all sizes. With the rise of cloud computing and AI-as-a-Service platforms, small and medium-sized enterprises can also leverage AI to improve operations, understand customers, and develop new products.
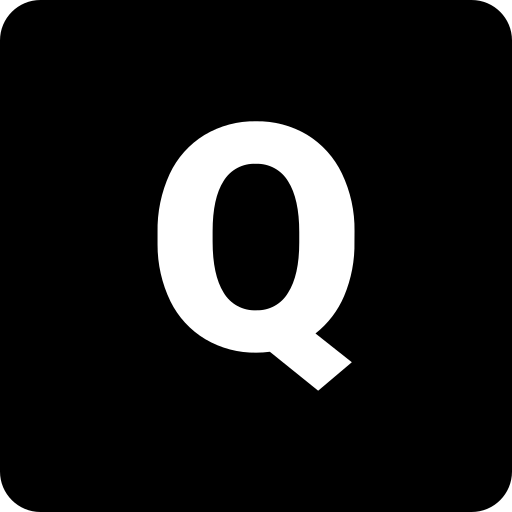
How can someone start learning about AI?
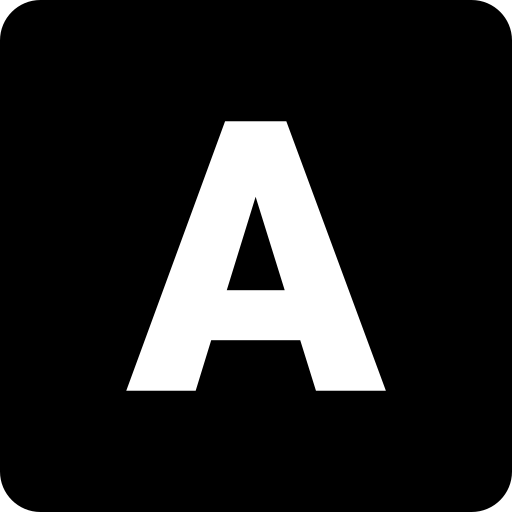
For those interested in AI, there are numerous online courses, tutorials, and resources available. Websites like Coursera, edX, and Khan Academy offer courses ranging from beginner to advanced levels. Additionally, many universities and tech companies provide free educational materials on AI.
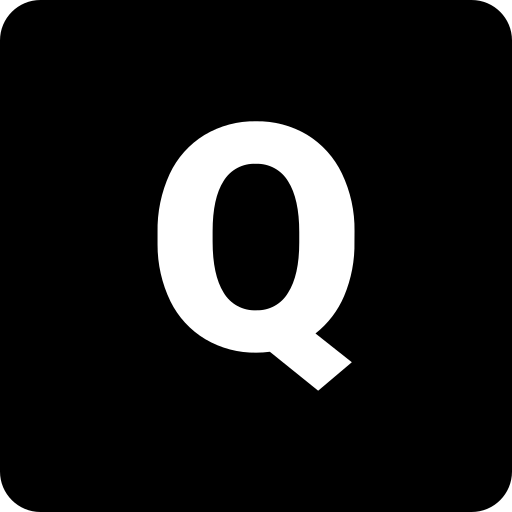
What are the most common AI applications we use daily?
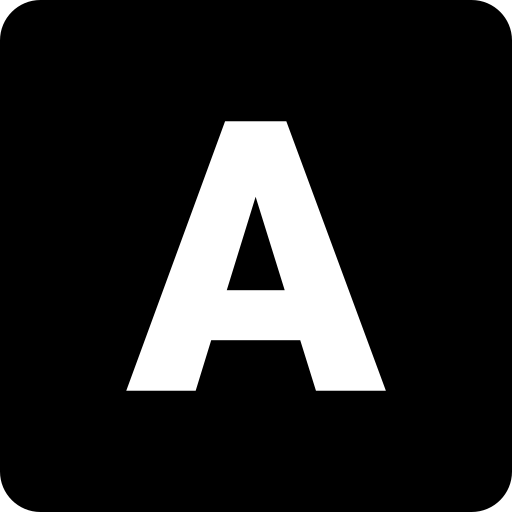
Common AI applications include virtual assistants (like Siri and Alexa), recommendation algorithms (such as those used by Netflix and Amazon), and navigation apps (like Google Maps). AI is also behind the scenes in search engines, social media feeds, and even in spam filters in your email.
Conclusion
Summary of Key Takeaways
Artificial Intelligence is transforming the world around us in profound ways. From everyday applications like smartphones and smart homes to advanced uses in healthcare and transportation, AI is making our lives easier and more efficient. Understanding the basics of AI helps us appreciate its potential and navigate the ethical and societal challenges it brings.
Final Thoughts on AI and Its Potential
As AI continues to evolve, it will undoubtedly play a larger role in shaping the future. Whether through solving global challenges, revolutionizing industries, or improving our daily lives, the potential of AI is vast. However, this potential must be harnessed responsibly, with attention to ethics, privacy, and inclusivity.